Machine Learning becomes an important part of every task in Visual Effects, Animation and games - including compositing. CopyCat is a Nuke node that “copies sequence-specific effects, such as garbage matting, beauty repairs, or deblurring, from a small number of frames in a sequence and then trains a network to replicate the effect on the entire sequence. CopyCat outputs a trained network in a .cat file, ready for the Inference node to apply your effect.”
CopyCat: Production
CopyCat could potentially simplify a production by merging different but similar shots in a sequence into one solution; do it once, repeat it on different shots.
Beauty work
Bruise/imperfection removal
Debeard (DC Studios spends $24 millions on reshoots including Henry Cavill's beard removal)
Garbage Matting
Clean-up
Markers removal
Strings removal
Upscaling
Deblurring
…
Why CopyCat?
Bruises removal shot with 340 frames.
Manual: 340 frames with 3 minutes/frame = 1020 min/17 hours
CopyCat: 2 frames with 5 minutes/frame = 120 min/2 hours
10 min corrections + 110 min training
Manual vs CopyCat: 15 hours or 89% time saving
Training time depends on the complexity, settings and hardware.
Training can be done on a different machine (similar to FX simulations).
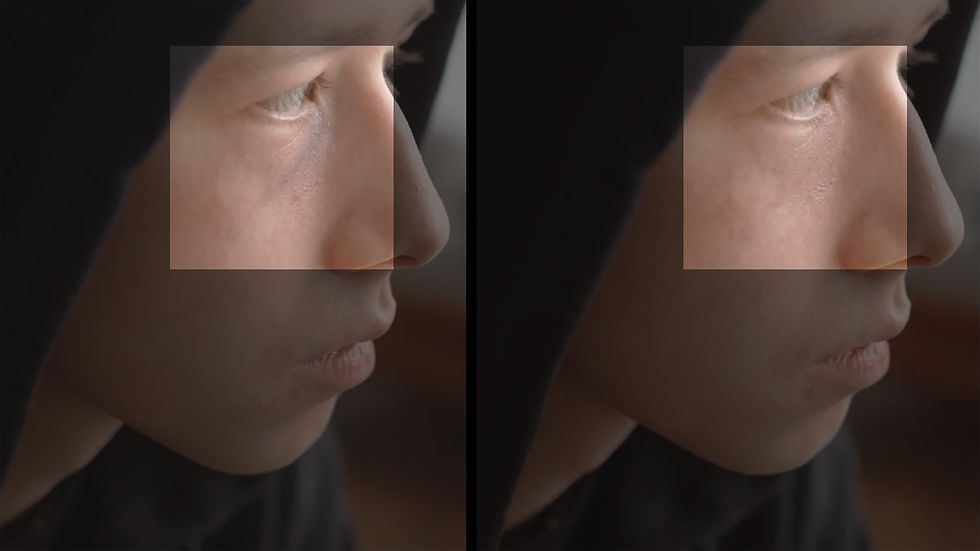
CopyCat x 21 Artist Show
We recently had an 21 Artist Show episode with Simon Devereux about accessing the Visual Effects industry. In the clip my recording equipment is clearly visible. My goal for this test was to take a short 386 frames clip and remove the headphones, microphone on my chest and the cable at the bottom of the frame.
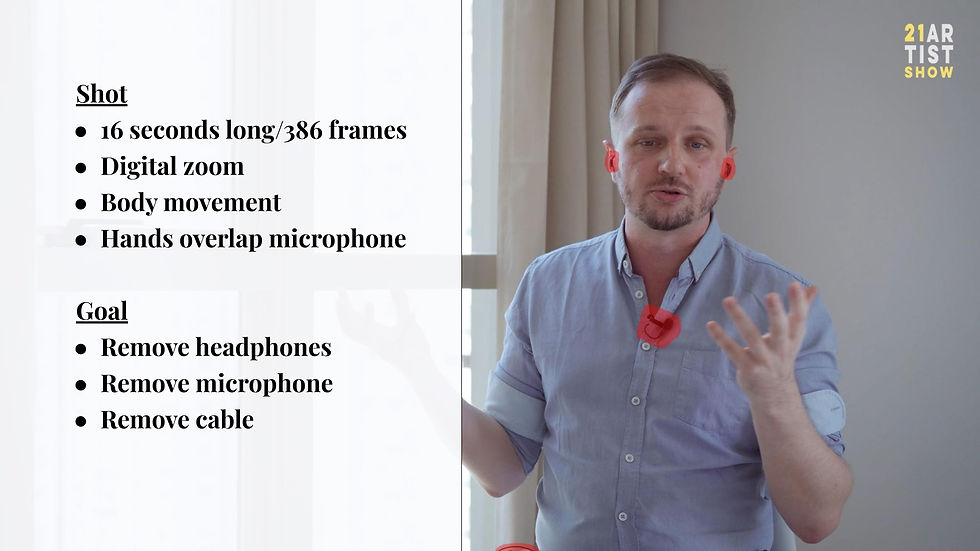
CopyCat v1
For the first training we only used one frame corrected in Photoshop with the generative fill. The total time for the test only took 30 minutes but with lacklaster results.
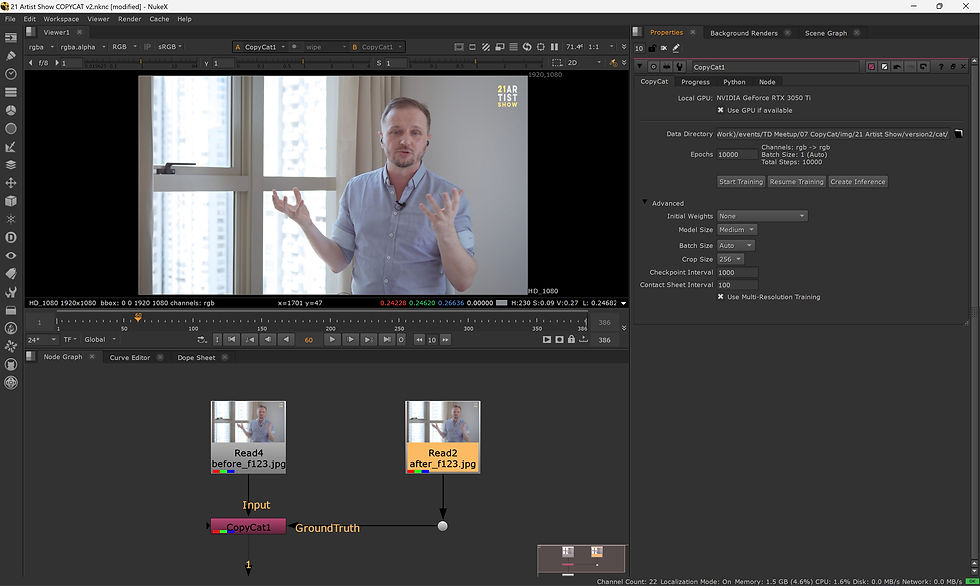
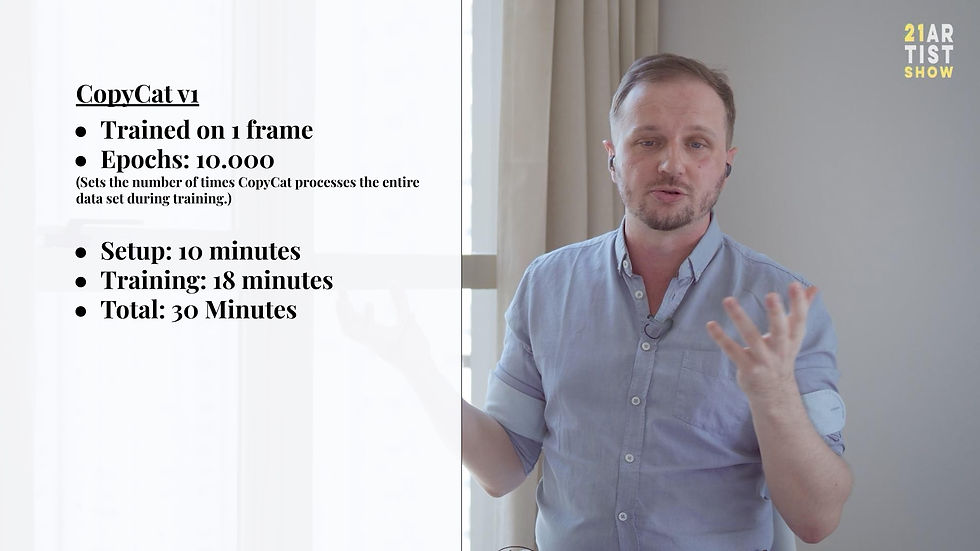
CopyCat v2
The 2nd version was about giving CopyCat more frames to work with while increasing the training time. The final result is 80% successful only failing when the hands brush over the microphone.
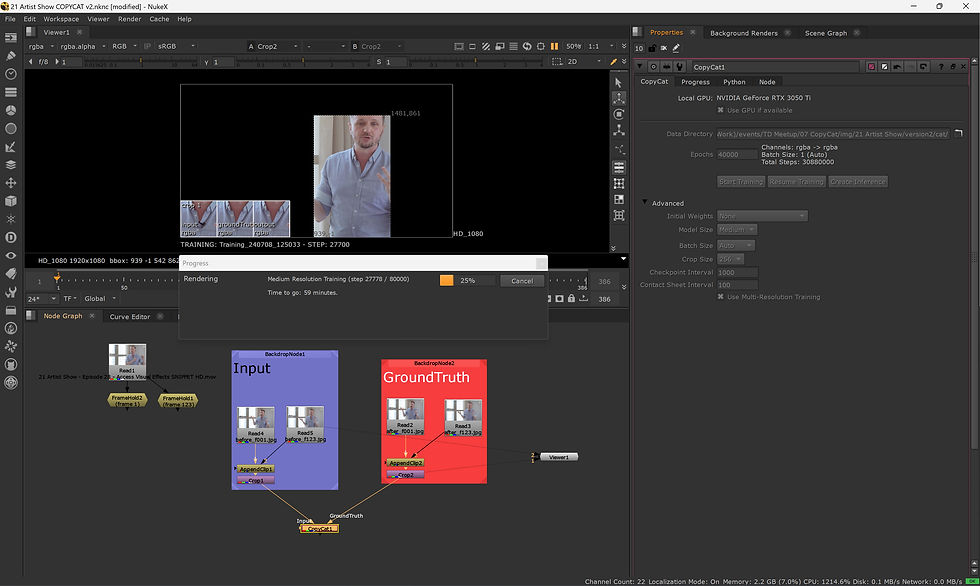
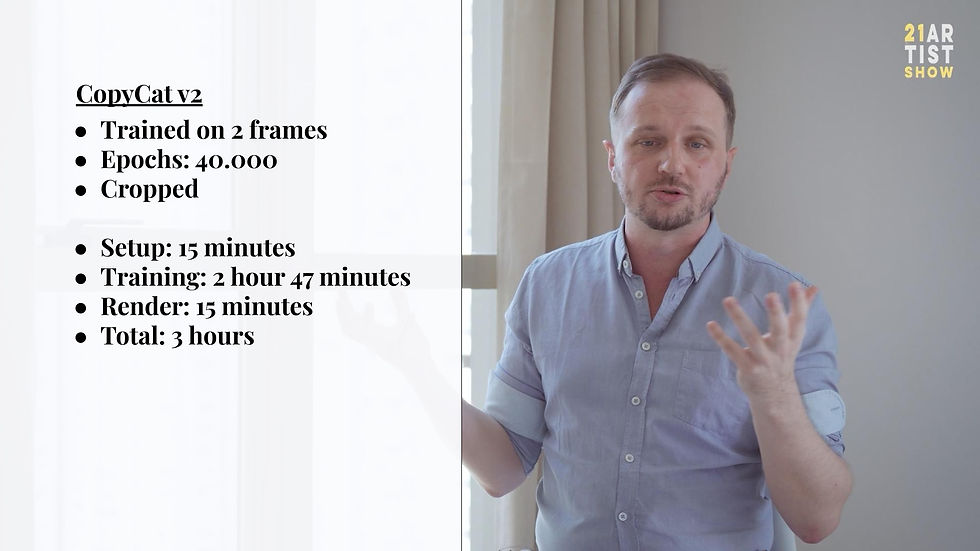
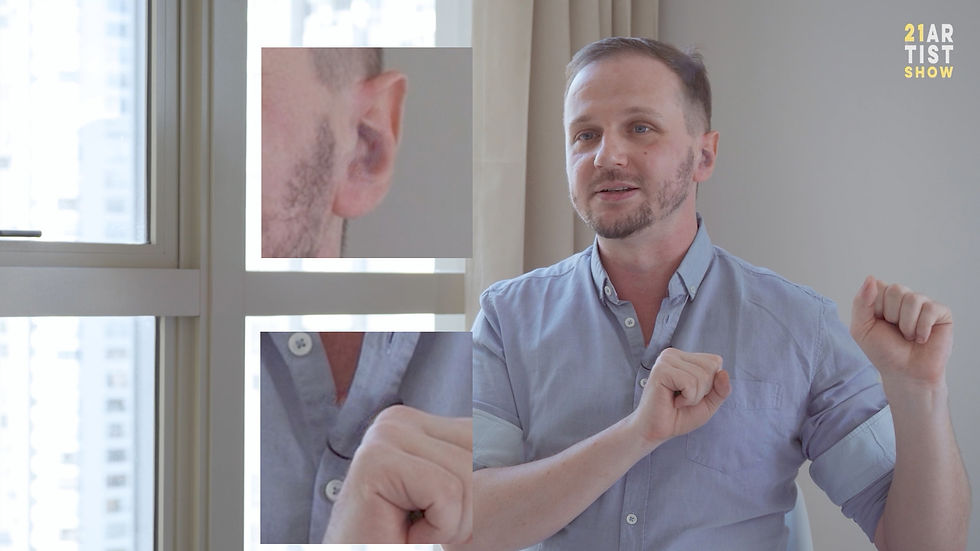
Resume
Learning to work with CopyCat was relatively easy thanks to its simple nature and the great masterclass from Foundry. Mastering CopyCat is a different beast and takes time and dedication. Nevertheless, CopyCat has the potential to speed up our compositing workflows while introducing us to machine learning in a controlled environment.
Give it a try and see if and how CopyCat can improve your compositing workflow.
Comentarios